
It is explored more deeply in (Spengler and Schiele, 2003) and (Shen et al., 2003). This method is proposed by Triesch and Malsburg in (Triesch and von der Malsburg, 2001). Democratic Integration is an architecture that allows the tracking of objects through the fusion of multiple adaptive cues in a self-organized fashion. In their works, different cues are combined directly through a likelihood manner however, a limitation of this method is that it does not take account of the cue’s discriminative ability. A straightforward approach is to use all cues in parallel and treat them as equivalent channels this approach has been reported in (Li and Francois, 2004) and (Wang and David, 2006). In this paper, we focus on the second key problem. The success of multi-cue based tracking algorithm relies on two key issues: (1) what features are used, (2) how the cues are integrated. Up to now, a number of literatures have been published about the fusion of multiple cues. Since most of the computer vision problems are ill-posed, more features increase the robustness of solutions. There are various features that can be used for representing objects, such as color, depth, motion and texture. To improve the robustness in tracking, multiple cues based methods has attracted the attention of researchers. In this case, the fixed feature is chose random, or, sometimes, preliminary experiments are runned to determine which feature to use. Though it is noted that the fusion of multiple cues will lead to an increased reliability of the tracking system, most of current tracking algorithms are based on single cue determined a priori and are, therefore, often limited to a particular environment. However, pedestrian tracking still suffers from a lack of robustness due to dynamic changing of the human body and the existing environment.
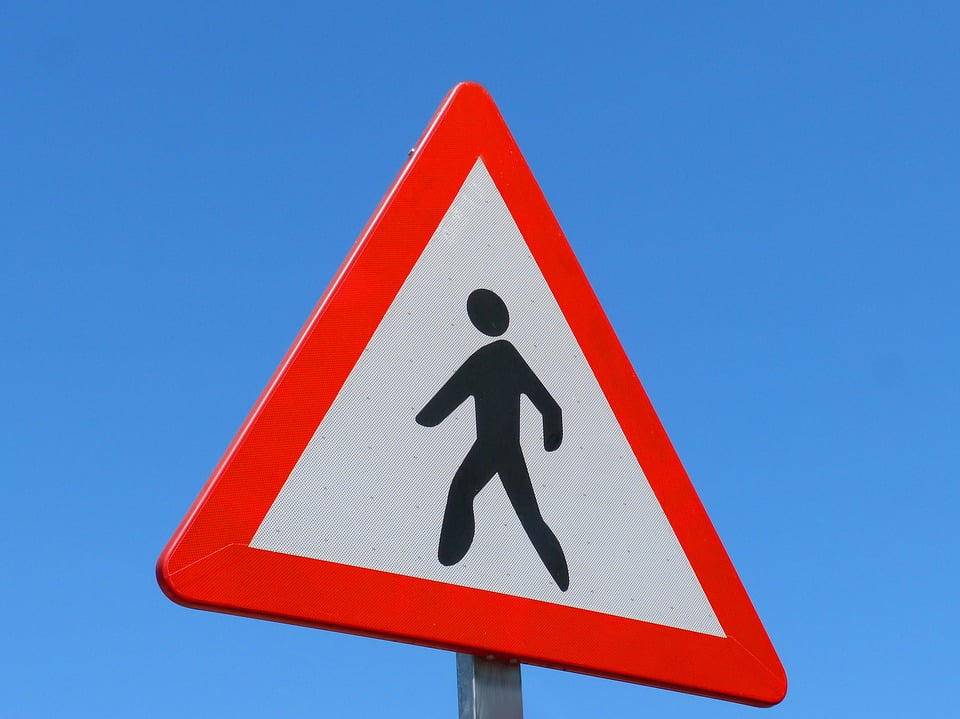
Great progress has been made as reported in the literatures. To achieve robustness and to reduce uncertainty in object racking, over the past few years tremendous research efforts have been devoted to the enhancement of visual tracking performance by designing various tracking programs and making use of different tracking features (Collins et al., 2005, Han and Davis, 2005, Yang et al., 2005, Comaniciu et al., 2000, Jepson et al., 2003, Comaniciu et al., 2003). With the exception of vision-based systems, some also use laser scanners to retrieve a 3Dmap of the terrain and detect pedestrians or uses ultrasonic sensors to determine the reflection of pedestrians. This method is very flexible however, the detecting performance heavily relies on the infrared imaging quality. (2004) first estimated the person candidate location through a “Projection-based” horizontal 49 segmentation and a “Brightness/Bodyline-based” vertical segmentation, then shape-independent features are applied to make a classification among those candidates. To solve the person detection problem, Fang et al. The limitation of this approach is that the stereo vision based system has less observing space than monocular vision based system.
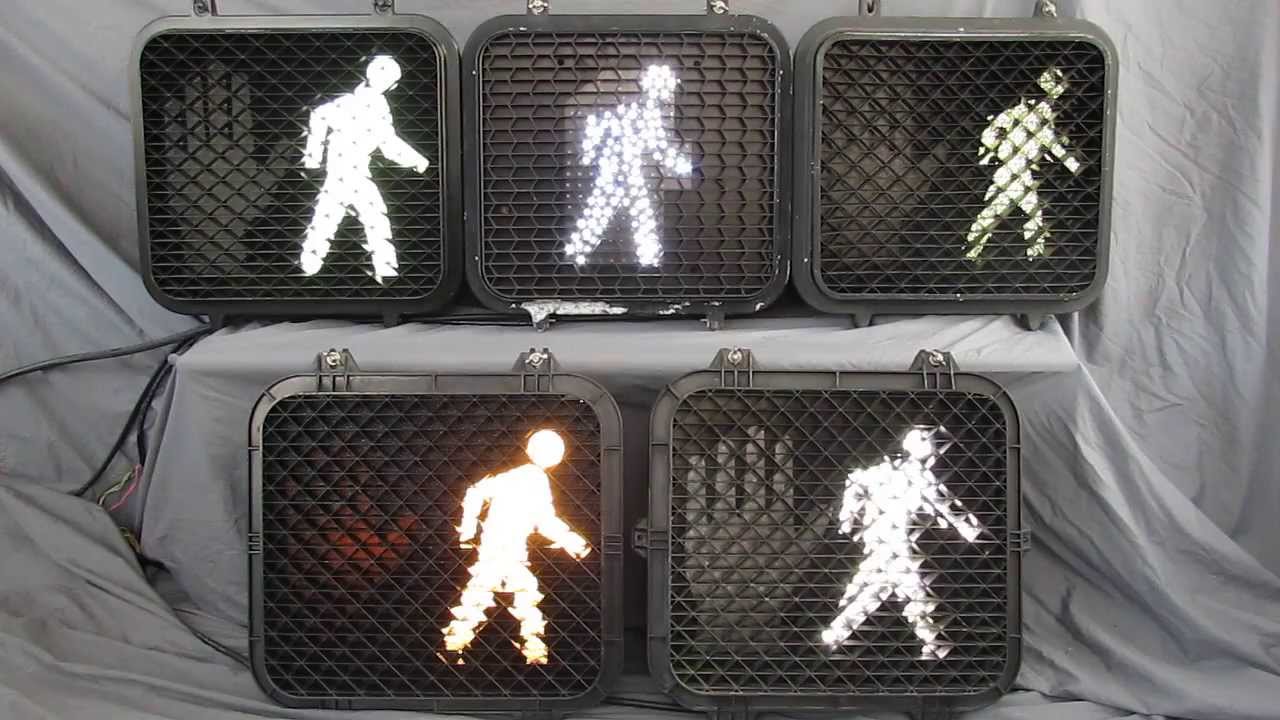
(2007) presents a stereo system for the detection of pedestrians using far-infrared cameras, in those system three detection technique: warm area detection, edge-based detection, and disparity computation are exploited according to different environmental conditions, based on this, a final validation process is performed using head morphological and thermal characteristics to confine the detecting result. (2007) presents a person detection method based on both shape and appearance cue, they first introduce a layered representation technique to separate the image into background and foreground layer, then the detection is solved as a multi scales template match problem base on the shape and appearance cues, this approach lead to high computation cost. Unfortunately pedestrian detection is a challenging task due to the non-rigidity of human body, and in the last decade, there have been many different approaches to solve this problem, like the use of monocular (Enzweiler and Gavrila, 2009), stereo vision (Bajracharya et al., 2009).

It is useful for many vision-based applications including visual surveillance, human–computer interfaces, traffic monitoring systems, video compression and many more. Pedestrian detection and tracking in video sequences is one of the main issues of computer vision.
